Machine learning in agriculture is an exciting and rapidly growing field. It has the potential to revolutionize the way we grow and produce food. Machine learning algorithms can help farmers optimize their operations and increase productivity, efficiency, and profitability by leveraging the power of artificial intelligence and data analytics.
What is Machine Learning in Agriculture?
Machine learning is a subset of artificial intelligence that involves the development of algorithms and models. It can learn from data and improve its performance without being explicitly programmed. In agriculture, you can apply machine learning to various tasks and applications. Including precision farming, pest and disease detection, crop yield prediction, irrigation management, and weather forecasting.
Here’s a survey on machine learning in agriculture: Machine learning in agriculture domain: A state-of-art survey
Benefits of Machine Learning in Agriculture
There are many benefits to using machine learning in agriculture. For farmers, machine learning can help improve decision-making by providing real-time data and insights. They can inform planting, irrigation, and fertilization strategies. It can also help identify patterns and trends in large data sets. Such as weather patterns or crop performance, which may be difficult for humans to discern. It can help farmers optimize their operations and increase productivity, efficiency, and profitability.
Precision Farming with Machine Learning
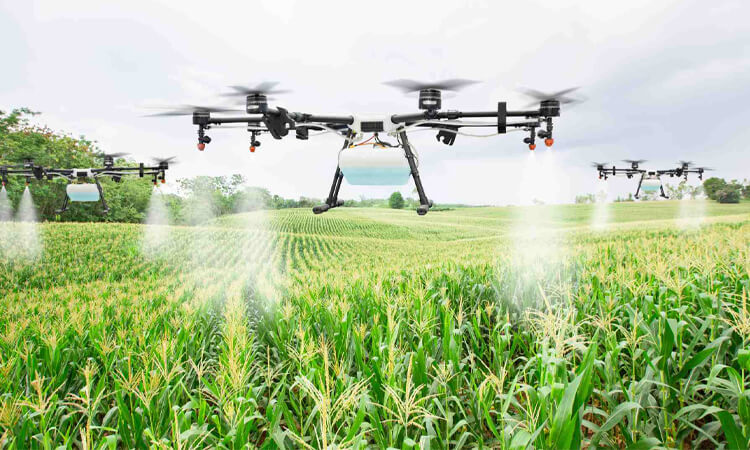
One of the most promising applications of machine learning in agriculture is precision farming. It involves using advanced technologies and data analytics to optimize crop production. It can include using sensors and other monitoring systems to gather data on soil conditions, weather, and crop performance. It can also use machine learning algorithms to analyze this data. You can also use this data to make informed irrigation, fertilization, and pest control decisions. Precision agriculture can help farmers reduce costs. It can also improve crop yields by efficiently using resources and targeting the most needed interventions.
Read more: Role of AI and Machine Learning in Robotics
Pest and Disease Detection with Machine Learning
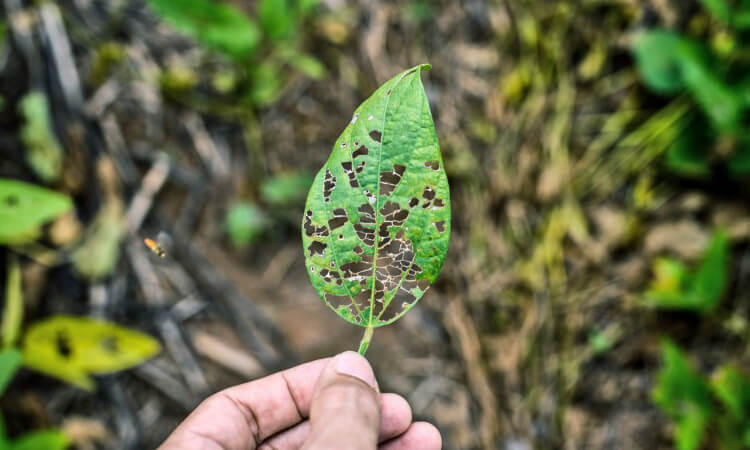
Pests and diseases can seriously affect crop yields and food security. And early detection and prevention is the key to minimizing their impact on farmers. Machine learning can help farmers detect pests and diseases earlier and more accurately. It analyzes data from sensors and cameras to identify signs of infestation or infection. It helps farmers take timely and targeted action to prevent or control disease outbreaks. It also helps reduce farmers’ need for expensive and potentially harmful chemicals.
Crop Yield Prediction with Machine Learning
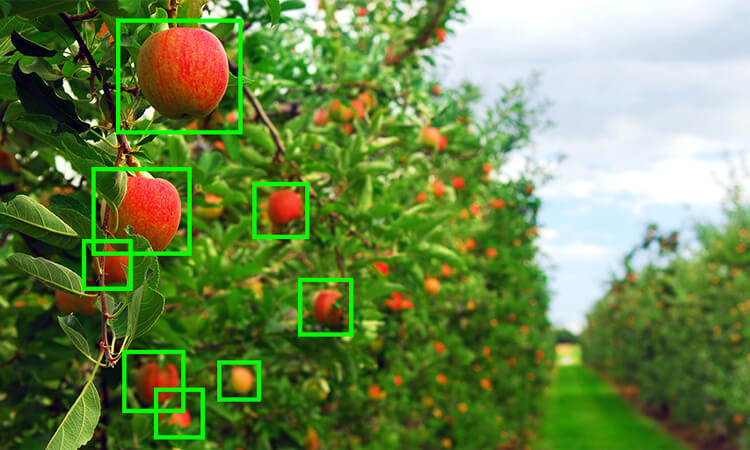
Forecasting crop yields is important to farmers. This is because it helps them plan for the future and makes informed decisions about planting and marketing. Machine learning can help improve crop yield predictions by analyzing weather, soil conditions, and previous crop performance by training machine learning models on large data sets. Farmers can develop more accurate and reliable yield forecasts. This data can help them plan for the future and optimize their operations.
Irrigation Management with Machine Learning
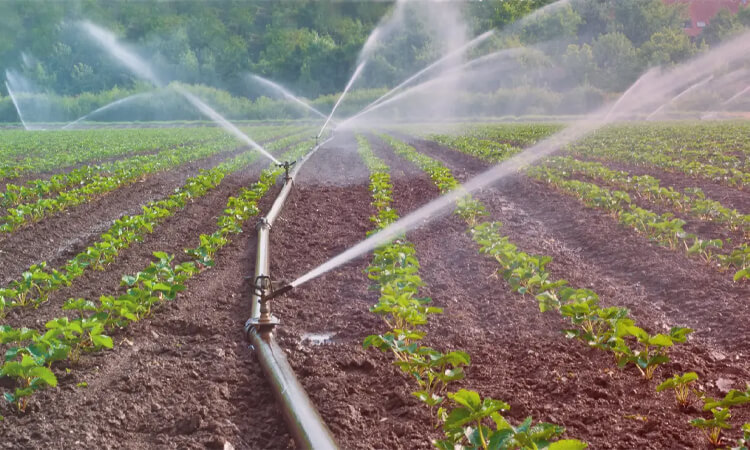
It is well known that water is an important resource for agriculture. Efficient irrigation management is the key to maximizing crop yields and minimizing water waste. Machine learning can analyze weather, soil moisture, and crop water requirements. The results of these analyses can help farmers optimize their irrigation practices. Farmers use machine learning to predict irrigation needs and optimize irrigation schedules. Farmers can conserve water and reduce costs while maintaining or increasing crop yields.
Supply Chain Management
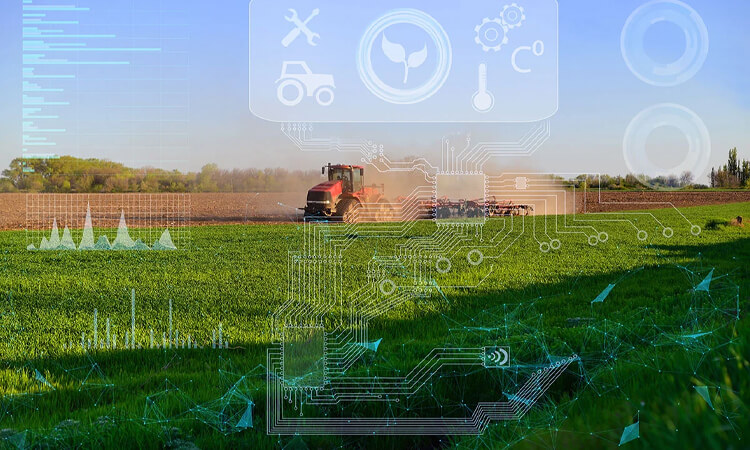
You also can use machine learning to optimize the supply chain in agriculture, from production to distribution. For example, a machine learning model might be able to analyze data on crop yields, demand, and transportation costs. You can use this data to optimize the routing and scheduling of deliveries. Farmers can reduce costs, increase efficiency and improve customer satisfaction by optimizing their supply chains.
Crop Monitoring
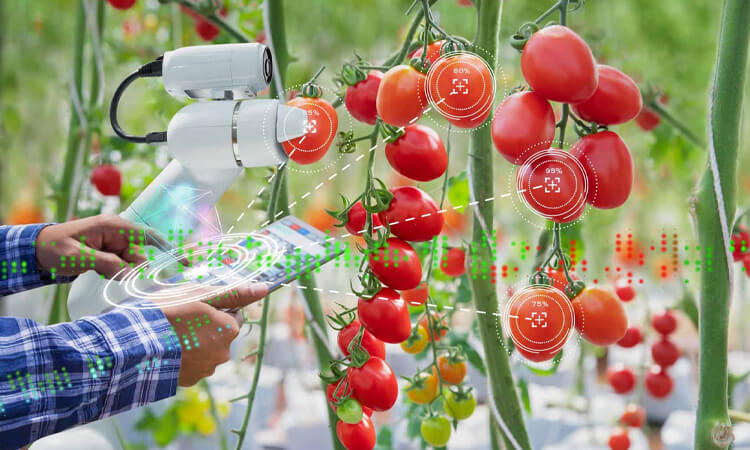
Crop monitoring is a key process for tracking crop growth and development. In this process, you can optimize production and identify any potential problems. It allows you to analyze the data collected from sensors, drones, and satellite images using machine learning algorithms. With this data, you can track crop growth and identify patterns and trends. It can help farmers make informed decisions. For example, a machine learning model may detect signs of pests and diseases. It can identify areas of a field that are experiencing drought stress. By monitoring crops more closely, farmers can take steps to address potential problems before they become major issues.
Weather Forecasting with Machine Learning
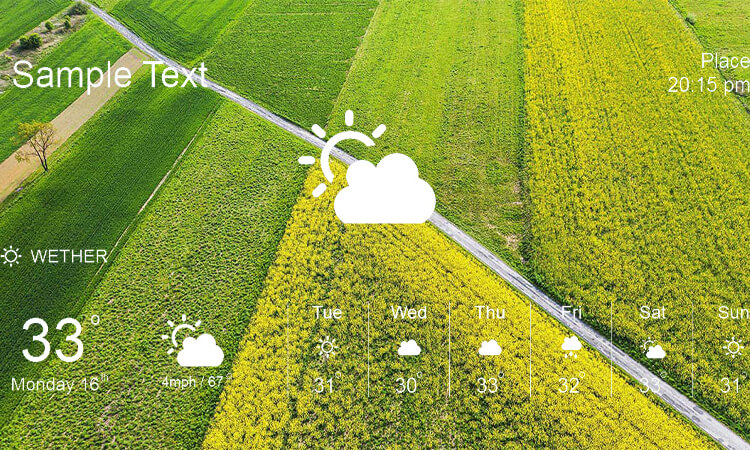
Weather plays a major role in agriculture, as it can significantly impact crop yields and farming operations. Machine learning algorithms can help farmers understand and predict weather patterns and trends better. This can optimize farming strategies. For example, machine learning models can analyze data from weather stations, satellites, and other sources. It can predict the likelihood of extreme weather events, such as droughts, floods, or frosts. By understanding the potential impact of weather on their operations, farmers can take steps to mitigate risk and protect their crops.
Automation Machine Learning in Agriculture
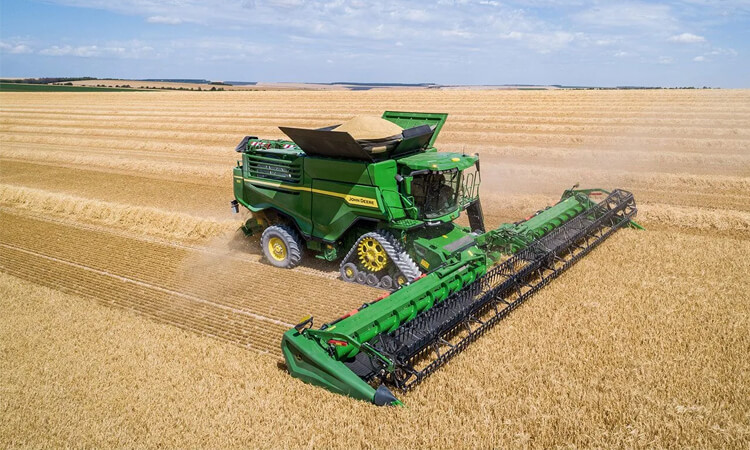
In addition to the applications mentioned above, you can use machine learning to automate a variety of tasks in agriculture. Some common ones are irrigation, seeding, and harvesting. For example, you can use machine learning algorithms to control irrigation systems. It will optimize the amount of water applied to different areas of the field based on factors such as soil moisture, weather patterns, and crop type. Similarly, machine learning can automatically sow seeds. It ensures that seeds are planted at the optimal depth and spacing for the largest yield. And in the future, you may be able to use machine learning to automate the harvesting process. You can also use robots or self-driving vehicles to pick and collect crops.
Applications of Machine Learning in Agriculture
There are many ways in which you can apply machine learning in agriculture to improve efficiency and productivity. Some of the most common applications include:
- Crop Yield Prediction: Machine learning can predict crop yields based on factors. Such as weather conditions, soil quality, and irrigation systems. It can help farmers make informed decisions about when to plant and harvest their crops, leading to increased productivity and profits.
- Pest and Disease Detection: Machine learning can identify pests and diseases in crops using visual data from cameras or sensors. It can help farmers take preventive measures to protect their crops, leading to fewer losses due to pest and disease outbreaks.
- Irrigation Systems: Machine learning can be used to optimize irrigation systems by analyzing data on weather patterns, soil moisture levels, and crop growth. It can help farmers use water more efficiently and reduce their water consumption.
Opportunities for Smallholder Farmers
While the application of machine learning in agriculture tends to focus on large-scale commercial operations. But there are opportunities for small farmers to enjoy this technology as well. Machine learning can help smallholder farmers optimize their operations and improve their productivity and profitability. This is despite the challenges they may face in terms of resources and access to technology. For example, Farm Together, an agricultural technology company, uses machine learning to analyze satellite and drone imagery. It helps small farmers optimize their irrigation and fertilization practices. Several initiatives and programs aim to bring machine learning technologies and expertise to smallholder farmers. One example is the national initiative “Digital Green.” It is an initiative that uses machine learning and other digital technologies to support the sustainable intensification of smallholder agriculture in developing countries.
Challenges in Implementing Machine Learning in Agriculture
While there are many benefits to using machine learning in agriculture. It also has some challenges that are difficult to solve. Some of the most significant challenges include the following:
- Access to Data: Data availability is one of the biggest challenges in implementing machine learning in agriculture. In many cases, much of the data we know is limited. Examples include data on weather patterns, soil conditions, and crop growth. Therefore it is difficult to train machine learning algorithms accurately.
- Infrastructure: Another challenge is the need for more infrastructure and resources in many rural areas, making it difficult to implement machine learning solutions. It includes electricity and internet connectivity, as well as the cost and availability of hardware and software.
- Cultural and Legal Issues: There are many issues to consider when implementing machine learning in agriculture. First, You must consider cultural and legal issues. In some cases, farmers may be concerned about the impact of new technologies on traditional farming practices. They may therefore be hesitant to adopt new technologies. There may also be legal issues surrounding the use of data and the ownership of intellectual property.
Ethical Considerations in Machine Learning in Agriculture
As with any technology, there are also ethical considerations to be taken into account when using machine learning in agriculture. One concern is the potential for machine learning algorithms to perpetuate or amplify existing biases. For example, racial or gender-biased information is present in the data they train. It can lead to unfair or discriminatory results. So it is important to ensure that machine learning algorithms are trained on diverse and representative datasets. This way, you can effectively reduce these risks. Another concern is that machine learning has the potential to automate or replace human work. These include the field of agriculture. You must also ensure that adopting machine learning does not lead to widespread job loss. It is used to augment and enhance human capabilities and improve working conditions.
The Future of Machine Learning in Agriculture
The future of machine learning in agriculture looks bright. It has many exciting possibilities waiting to be explored. Machine learning is a key area of research for researchers. Machine learning improves crop breeding and genetic improvement by analyzing large crop genetics and performance datasets. Another area of focus is analyzing demand and supply patterns, transportation networks, and market conditions. We can use machine learning to optimize supply chains and logistics. Machine learning can also be used by analyzing data on food processing and handling methods. We can also use it to identify potential risks or contaminants to improve food safety and quality. As machine learning technology continues to evolve and become more widespread, we expect to see more applications in agriculture. This technology’s development brings us closer to a more sustainable and productive food system.
Agriculture with machine learning is revolutionizing the agricultural industry. It enables farmers to make smarter decisions, optimize resource allocation, and increase crop yields. As technology evolves, we can expect more innovative applications of machine learning in agriculture. While further improving the efficiency and sustainability of modern agricultural practices.
About Machine Learning in Agriculture FAQs
-
What is machine learning, and how is it being used in agriculture?
Machine learning is artificial intelligence that allows machines to learn and improve from experience without being explicitly programmed. In agriculture, machine learning is used to analyze vast amounts of data to improve crop yields, predict weather patterns, identify pests and diseases, and more.
-
How can machine learning algorithms be trained to identify pests and diseases in crops?
Machine learning algorithms can be trained to identify pests and diseases in crops by analyzing large datasets of labeled images of healthy and diseased plants. This allows the algorithms to learn to recognize patterns and identify potential issues before they become widespread.
-
What data is needed for machine learning to be effective in agriculture?
Machine learning algorithms need access to high-quality data to be effective. This includes historical weather data, soil data, crop yield data, and more. Then, people can use this data to train algorithms to make accurate predictions and recommendations.
-
Can machine learning help farmers optimize crop yields and reduce waste?
Yes, machine learning can help farmers optimize crop yields and reduce waste by analyzing soil quality, weather patterns, and crop growth data to identify the most effective planting strategies and management techniques.
-
How can machine learning be used for precision agriculture, such as irrigation and fertilizer management?
Machine learning can be used for precision agriculture by analyzing data from sensors and other sources to provide real-time insights into soil quality, moisture levels, and nutrient content. Then, this can be used to optimize irrigation and fertilizer management, reducing waste and improving crop yields.
-
How can machine learning help predict weather patterns and their impact on crops?
Machine learning can help predict weather patterns and their impact on crops by analyzing historical weather data and using that information to predict future weather patterns. Then, these predictions can be used to make informed decisions about planting strategies and crop management techniques.
-
How can machine learning be combined with other technologies, such as drones and sensors, to improve agricultural outcomes?
Machine learning can be combined with other technologies to improve agricultural outcomes by providing real-time data on crop health, soil quality, and more, such as drones and sensors. This data can then be used to make informed decisions about crop management and improve overall agricultural efficiency.